Book Downloads Hub Reads Ebooks Online eBook Librarys Digital Books Store Download Book Pdfs Bookworm Downloads Book Library Help Epub Book Collection Pdf Book Vault Read and Download Books Open Source Book Library Best Book Downloads David Arnett Cara Brookins Jamie Campbell Kim Chernin Erasmo Gamboa Peter Brandvold Gerald Schloemer Janine Myung Ja
Do you want to contribute by writing guest posts on this blog?
Please contact us and send us a resume of previous articles that you have written.
Understanding Stochastic Dynamic Programming: Unveiling the Mathematical Probability

Stochastic dynamic programming is a powerful mathematical framework used in diverse fields such as economics, finance, engineering, and computer science. It helps us make optimal decisions in uncertain and dynamic environments, where future outcomes are influenced by both random factors and our actions. In this article, we will explore the fundamentals of stochastic dynamic programming, its applications, and how mathematical probability plays a crucial role in this domain.
What is Stochastic Dynamic Programming?
Stochastic dynamic programming is a branch of mathematical optimization that deals with sequential decision-making under uncertainty. Unlike traditional dynamic programming, which assumes deterministic transitions between states, stochastic dynamic programming accounts for the randomness inherent in real-world situations.
The core idea behind stochastic dynamic programming is to formulate a problem as a sequence of decisions made over time, where the current decision impacts future states and outcomes. By considering the uncertainty in the future, the objective is to find the optimal decision policy that maximizes or minimizes an expected objective function.
4.3 out of 5
Language | : | English |
File size | : | 8692 KB |
Print length | : | 164 pages |
Screen Reader | : | Supported |
X-Ray for textbooks | : | Enabled |
The Components of Stochastic Dynamic Programming
Stochastic dynamic programming involves several key components that influence the decision-making process:
- States: A state represents the current condition or situation from which decisions are made. States can be discrete or continuous, and they capture all relevant information needed to make decisions.
- Actions: Actions refer to the available choices or decisions that can be made at each state. For example, in a financial portfolio management problem, actions could be buying or selling certain stocks.
- Transition Probabilities: Transition probabilities describe the likelihood of moving from one state to another after taking a specific action. These probabilities capture the randomness and uncertainty in the system.
- Rewards or Costs: Rewards or costs quantify the desirability or undesirability of being in a certain state or taking a particular action. They influence the objective function that needs to be optimized.
- Discount Factor: The discount factor represents the trade-off between current and future rewards. A higher discount factor assigns more importance to near-term rewards, while a lower discount factor emphasizes long-term gains.
Applications of Stochastic Dynamic Programming
Stochastic dynamic programming finds numerous applications across different domains. Some prominent examples include:
- Inventory Management: Determining optimal order policies for perishable goods, considering uncertain demand patterns.
- Financial Portfolio Optimization: Designing investment strategies that balance risk and return under uncertain market conditions.
- Energy Resource Allocation: Finding the optimal allocation of limited resources across different energy generation units to minimize costs and maximize efficiency.
- Supply Chain Optimization: Optimizing production, inventory, and distribution decisions to maximize profitability while accounting for uncertain demand, supply, and transportation constraints.
The Role of Mathematical Probability
Mathematical probability plays a crucial role in stochastic dynamic programming. It helps us model the uncertainty present in the system and quantify the likelihood of various future outcomes.
With the help of probability distributions, we can assign probabilities to different states, actions, and outcomes. These probabilities enable us to compute the expected values of rewards and costs, allowing us to make optimal decisions based on the objective function.
Furthermore, probability theory provides the foundation for analyzing the properties and characteristics of stochastic dynamic programming models. It enables us to study convergence properties, stability, and sensitivity to changes in model parameters.
Stochastic dynamic programming is a powerful mathematical framework that revolutionizes decision-making under uncertainty. By considering probabilistic transitions between states and outcomes, it allows us to find optimal solutions across diverse application areas.
Understanding the core components of stochastic dynamic programming, its applications, and the role of mathematical probability empowers us to tackle complex decision problems and optimize outcomes effectively. So dive into this fascinating field and unlock the potential of stochastic dynamic programming!
4.3 out of 5
Language | : | English |
File size | : | 8692 KB |
Print length | : | 164 pages |
Screen Reader | : | Supported |
X-Ray for textbooks | : | Enabled |
to Stochastic Dynamic Programming presents the basic theory and examines the scope of applications of stochastic dynamic programming. The book begins with a chapter on various finite-stage models, illustrating the wide range of applications of stochastic dynamic programming. Subsequent chapters study infinite-stage models: discounting future returns, minimizing nonnegative costs, maximizing nonnegative returns, and maximizing the long-run average return. Each of these chapters first considers whether an optimal policy need exist—providing counterexamples where appropriate—and then presents methods for obtaining such policies when they do. In addition, general areas of application are presented. The final two chapters are concerned with more specialized models. These include stochastic scheduling models and a type of process known as a multiproject bandit. The mathematical prerequisites for this text are relatively few. No prior knowledge of dynamic programming is assumed and only a moderate familiarity with probability— including the use of conditional expectation—is necessary.
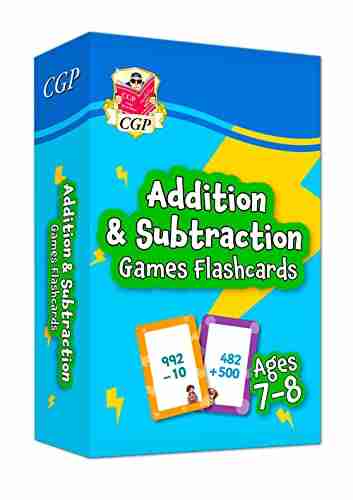

The Ultimate Guide to New Addition Subtraction Games...
In this day and age, countless parents are...
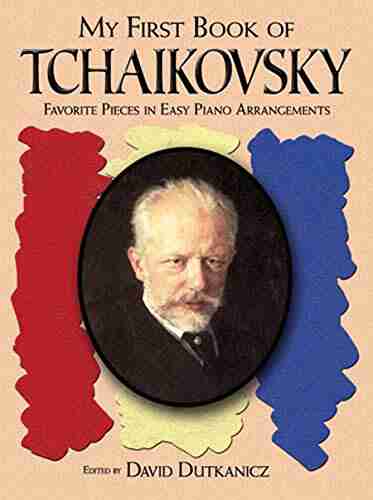

The Ultimate Guide for the Aspiring Pianist: Unleash Your...
Are you a beginner pianist feeling...
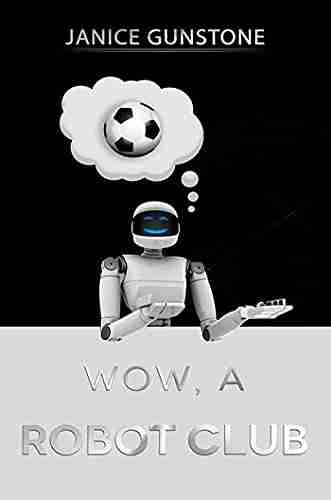

Wow Robot Club Janice Gunstone - The Mastermind Behind...
Robots have always fascinated...
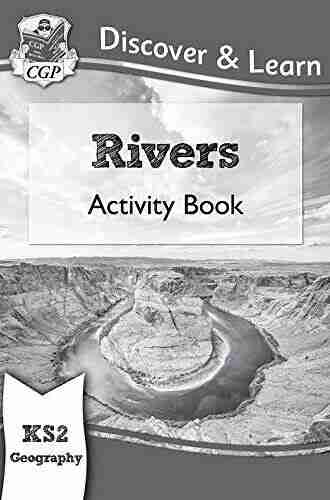

Ideal For Catching Up At Home: CGP KS2 Geography
Are you looking for the perfect resource to...
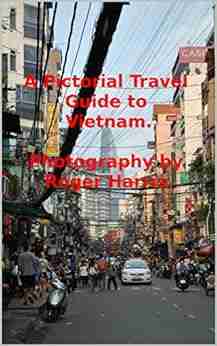

The Ultimate Pictorial Travel Guide To Vietnam: Explore...
Discover the rich...
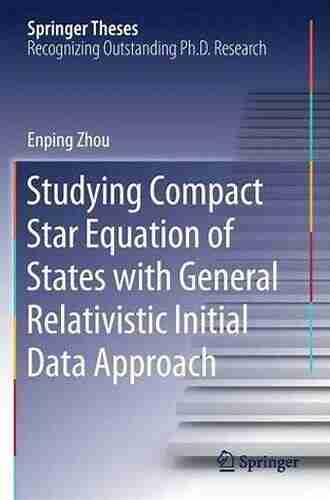

Unlocking the Secrets of Compact Stars: Exploring...
Compact stars have...
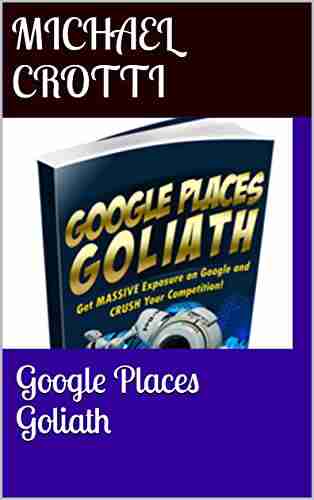

Unveiling the Hidden Gem: Google Places Goliath Valley...
Are you tired of visiting the same old...
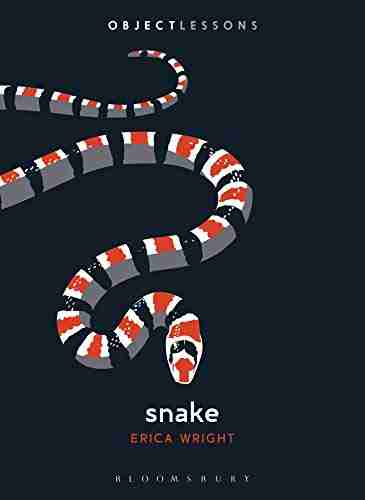

Essays Towards Theory Of Knowledge: Exploring the Depths...
Are you ready to delve into...
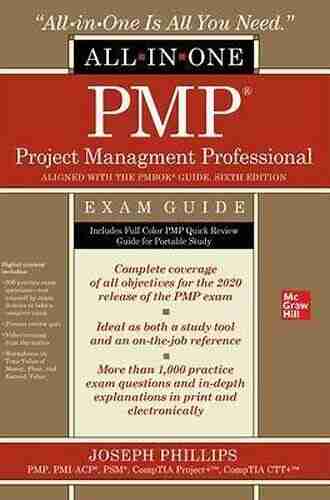

The Ultimate PMP Project Management Professional All In...
Are you ready to take your project...
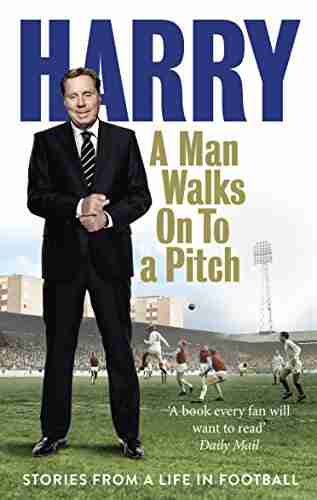

10 Incredible Stories From Life In Football That Will...
The Beautiful Game - Football...
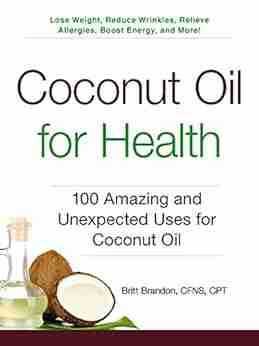

100 Amazing And Unexpected Uses For Coconut Oil
Coconut oil, a versatile and widely loved...
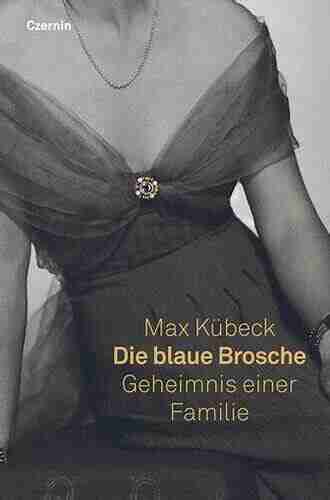

Unveiling the Enigma of Die Blaue Brosche: A Family’s...
Have you ever heard of Die Blaue Brosche...
Light bulbAdvertise smarter! Our strategic ad space ensures maximum exposure. Reserve your spot today!
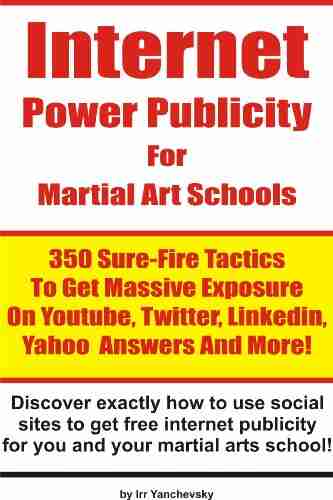

- Frank ButlerFollow ·3.3k
- Russell MitchellFollow ·6.8k
- Gustavo CoxFollow ·19.1k
- Mark TwainFollow ·2.4k
- Fernando PessoaFollow ·7.2k
- Stuart BlairFollow ·12.5k
- Bradley DixonFollow ·10.8k
- Herb SimmonsFollow ·6k