Book Downloads Hub Reads Ebooks Online eBook Librarys Digital Books Store Download Book Pdfs Bookworm Downloads Book Library Help Epub Book Collection Pdf Book Vault Read and Download Books Open Source Book Library Best Book Downloads Shelley Wicks Sarah Wynde David Searcy Barbara Slavin Bring On Fitness Cristiano Rizzi Jamal Omar Soseh Yekanians
Do you want to contribute by writing guest posts on this blog?
Please contact us and send us a resume of previous articles that you have written.
Feature Engineering Made Easy: The Ultimate Guide

Feature engineering is a critical step in the machine learning pipeline. It involves transforming raw data into a format that is more suitable for modeling. While it is an essential process, it can also be complex and time-consuming. Fortunately, with the right tools and techniques, feature engineering can be made easy, allowing you to focus more on building powerful machine learning models. In this ultimate guide, we will dive deep into feature engineering and provide you with practical tips and tricks to make the process a breeze.
What is Feature Engineering?
Feature engineering is the process of creating new features from existing data to improve model performance. It involves selecting, creating, and transforming variables in the dataset to make them more informative and relevant for the machine learning algorithm. The goal is to extract meaningful patterns and relationships from the data that the model can use to make accurate predictions.
Why is Feature Engineering Important?
Feature engineering plays a crucial role in machine learning for several reasons:
4.3 out of 5
Language | : | English |
File size | : | 8251 KB |
Text-to-Speech | : | Enabled |
Screen Reader | : | Supported |
Enhanced typesetting | : | Enabled |
Print length | : | 318 pages |
- Improved Predictive Performance: By engineering meaningful features, you can enhance the predictive power of your models.
- Reduced Dimensionality: By eliminating redundant or irrelevant features, you can reduce the dimensionality of the dataset, leading to faster training and improved model interpretability.
- Dealing with Missing Values: Feature engineering techniques can help you handle missing values in a meaningful way, preventing loss of important information.
- Enhanced Model Robustness: By transforming variables, you can make your model more robust to outliers and noise in the data.
Popular Feature Engineering Techniques
There are numerous feature engineering techniques that can be used depending on the nature of the data and the problem at hand. Here are some of the most popular ones:
One-hot Encoding
This technique is used to convert categorical variables into a binary representation. It creates new binary columns, one for each unique value of the categorical variable, indicating the presence or absence of that value in the original data.
Feature Scaling
Feature scaling is a process of standardizing numerical features to a common scale. This ensures that all features contribute equally to the model and prevents bias towards variables with larger magnitudes.
Polynomial Features
Polynomial features involve creating new features by raising existing features to a certain power. This technique captures non-linear relationships between variables and can improve model performance.
Tips for Easy Feature Engineering
While feature engineering can seem overwhelming, following these tips can make the process easier and more effective:
Understand Your Data
Before diving into feature engineering, it is crucial to have a deep understanding of your data. Explore its distribution, identify outliers, and gain insights into the relationships between variables. This will guide you in selecting the right techniques for feature engineering.
Use Domain Knowledge
Domain knowledge can be a powerful tool when it comes to feature engineering. Incorporate your understanding of the problem domain to create features that capture the relevant information and patterns. This can significantly improve model performance.
Automate the Process
Take advantage of available tools and libraries to automate feature engineering tasks. There are several Python libraries, such as scikit-learn and Featuretools, that provide pre-built functions for common feature engineering tasks. This can save you time and effort in creating features from scratch.
Feature engineering is a crucial step in the machine learning pipeline and can greatly influence model performance. By understanding the data, using domain knowledge, and leveraging automated tools, feature engineering can be made easy and effective. Remember to experiment with different techniques and iterate on your feature engineering process to find the best set of features for your models. With these tips and tricks, you are now equipped to tackle feature engineering with ease. Happy modeling!
4.3 out of 5
Language | : | English |
File size | : | 8251 KB |
Text-to-Speech | : | Enabled |
Screen Reader | : | Supported |
Enhanced typesetting | : | Enabled |
Print length | : | 318 pages |
A perfect guide to speed up the predicting power of machine learning algorithms
Key Features
- Design, discover, and create dynamic, efficient features for your machine learning application
- Understand your data in-depth and derive astonishing data insights with the help of this Guide
- Grasp powerful feature-engineering techniques and build machine learning systems
Book Description
Feature engineering is the most important step in creating powerful machine learning systems. This book will take you through the entire feature-engineering journey to make your machine learning much more systematic and effective.
You will start with understanding your data—often the success of your ML models depends on how you leverage different feature types, such as continuous, categorical, and more, You will learn when to include a feature, when to omit it, and why, all by understanding error analysis and the acceptability of your models. You will learn to convert a problem statement into useful new features. You will learn to deliver features driven by business needs as well as mathematical insights. You'll also learn how to use machine learning on your machines, automatically learning amazing features for your data.
By the end of the book, you will become proficient in Feature Selection, Feature Learning, and Feature Optimization.
What you will learn
- Identify and leverage different feature types
- Clean features in data to improve predictive power
- Understand why and how to perform feature selection, and model error analysis
- Leverage domain knowledge to construct new features
- Deliver features based on mathematical insights
- Use machine-learning algorithms to construct features
- Master feature engineering and optimization
- Harness feature engineering for real world applications through a structured case study
Who this book is for
If you are a data science professional or a machine learning engineer looking to strengthen your predictive analytics model, then this book is a perfect guide for you. Some basic understanding of the machine learning concepts and Python scripting would be enough to get started with this book.
Table of Contents
- to Feature Engineering
- Feature Understanding - What’s in My Data?
- Feature Improvement - Cleaning Datasets
- Feature Construction
- Feature Selection
- Feature Transformations
- Automatic Construction of Features
- Case Studies
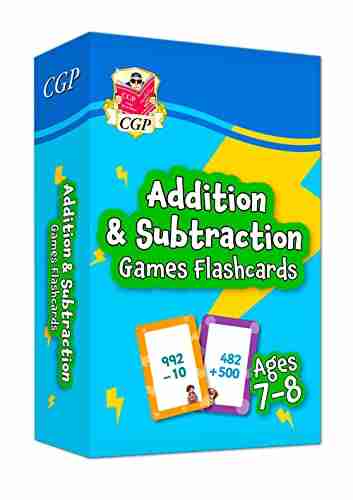

The Ultimate Guide to New Addition Subtraction Games...
In this day and age, countless parents are...
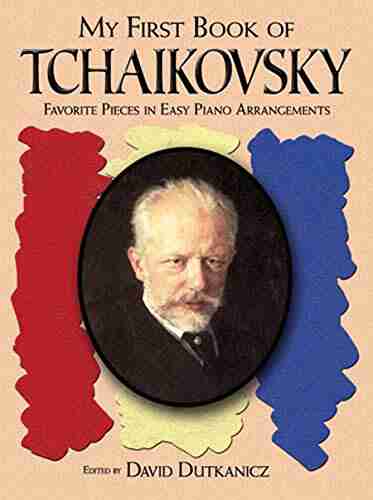

The Ultimate Guide for the Aspiring Pianist: Unleash Your...
Are you a beginner pianist feeling...
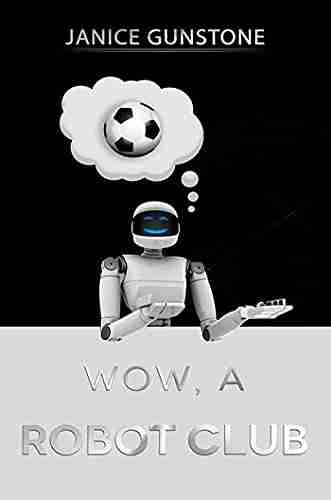

Wow Robot Club Janice Gunstone - The Mastermind Behind...
Robots have always fascinated...
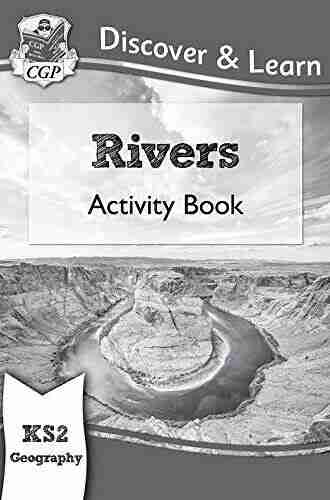

Ideal For Catching Up At Home: CGP KS2 Geography
Are you looking for the perfect resource to...
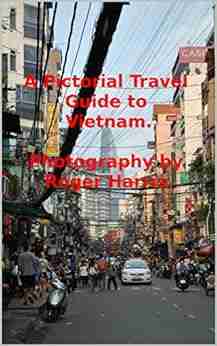

The Ultimate Pictorial Travel Guide To Vietnam: Explore...
Discover the rich...
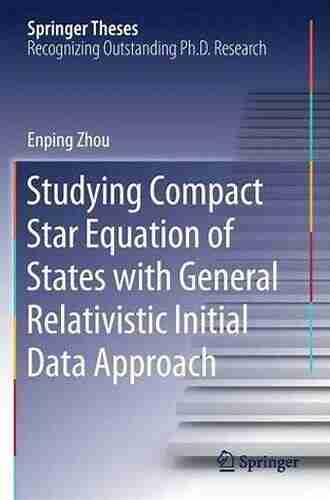

Unlocking the Secrets of Compact Stars: Exploring...
Compact stars have...
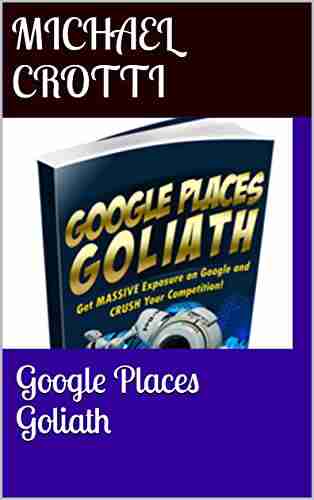

Unveiling the Hidden Gem: Google Places Goliath Valley...
Are you tired of visiting the same old...
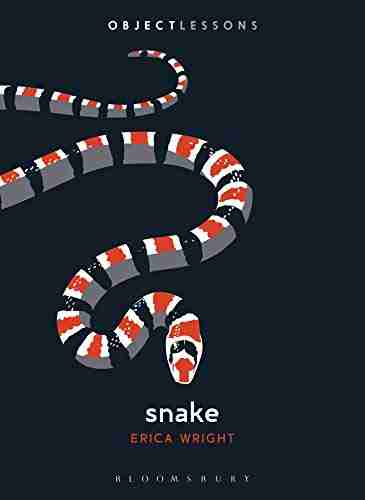

Essays Towards Theory Of Knowledge: Exploring the Depths...
Are you ready to delve into...
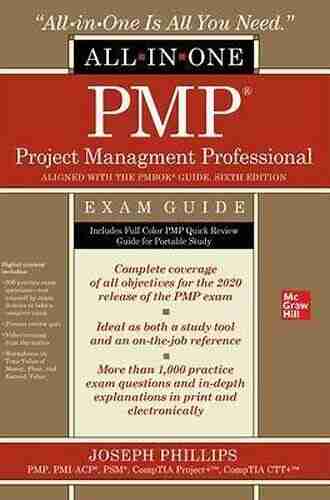

The Ultimate PMP Project Management Professional All In...
Are you ready to take your project...
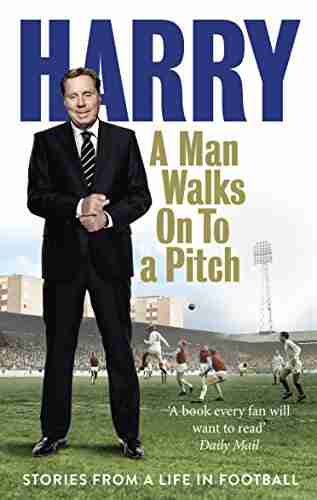

10 Incredible Stories From Life In Football That Will...
The Beautiful Game - Football...
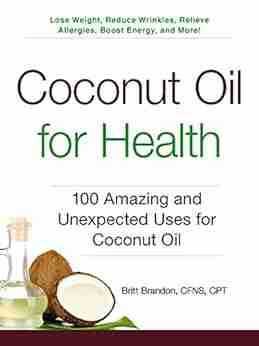

100 Amazing And Unexpected Uses For Coconut Oil
Coconut oil, a versatile and widely loved...
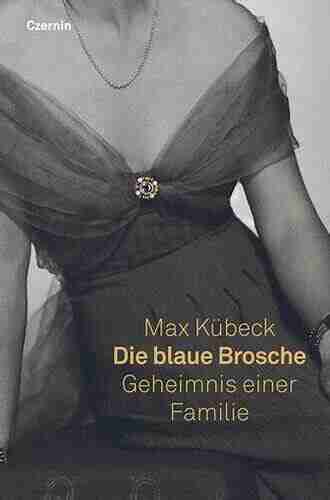

Unveiling the Enigma of Die Blaue Brosche: A Family’s...
Have you ever heard of Die Blaue Brosche...
Light bulbAdvertise smarter! Our strategic ad space ensures maximum exposure. Reserve your spot today!
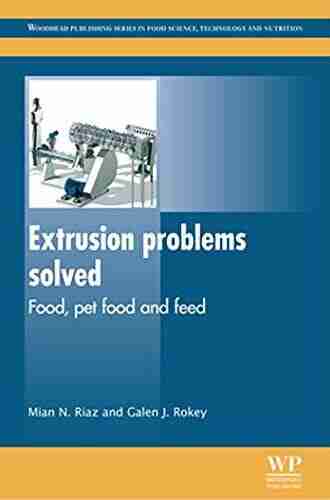

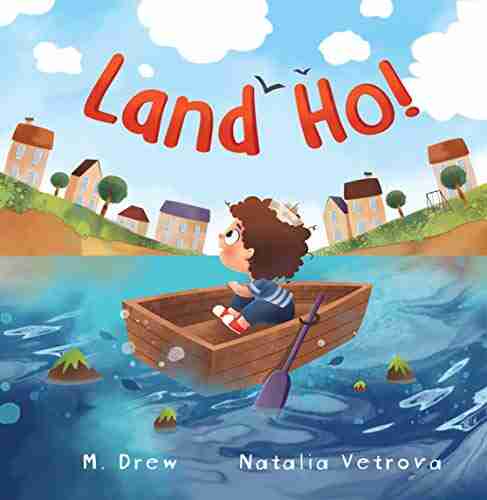

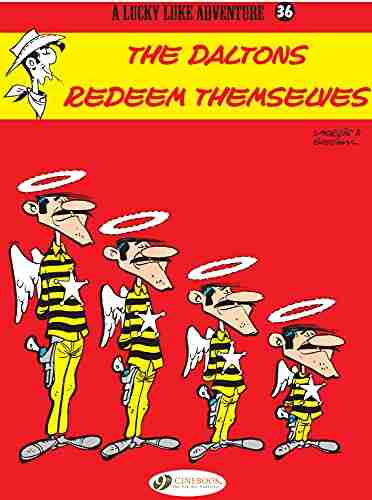

- Roberto BolañoFollow ·9.3k
- Jan MitchellFollow ·4k
- Camden MitchellFollow ·5k
- Eli BlairFollow ·11k
- J.D. SalingerFollow ·2.3k
- Mark TwainFollow ·2.4k
- Logan CoxFollow ·8.1k
- Victor HugoFollow ·3.2k