Book Downloads Hub Reads Ebooks Online eBook Librarys Digital Books Store Download Book Pdfs Bookworm Downloads Book Library Help Epub Book Collection Pdf Book Vault Read and Download Books Open Source Book Library Best Book Downloads Joanne Hayle Robin Catling Ken Davenport Lars Horn Maris Wicks Jackie Speicher J S Rowlinson Vassilios Mcinnes Spathopoulos
Do you want to contribute by writing guest posts on this blog?
Please contact us and send us a resume of previous articles that you have written.
Marketing Analysis And Prediction Using Machine Learning And Deep Learning

In today's digital age, companies have access to vast amounts of data that can provide valuable insights into consumer behavior and preferences. Marketing teams are constantly looking for ways to effectively analyze this data and predict future trends, in order to make informed decisions and stay ahead of the competition. With the advancements in machine learning and deep learning, marketers now have powerful tools at their disposal to extract meaningful patterns from data and make accurate predictions.
The Power of Machine Learning in Marketing
Machine learning is a subset of artificial intelligence that focuses on developing algorithms that can learn from and make predictions or decisions based on data. In marketing, machine learning algorithms can analyze large datasets and identify patterns, relationships, and trends that human analysts may miss. This enables marketing teams to gain a deeper understanding of customer preferences, purchase behavior, and market trends.
One of the key applications of machine learning in marketing is customer segmentation. By analyzing various factors such as demographics, purchasing habits, and browsing behavior, machine learning algorithms can group customers into segments with similar characteristics. This allows marketers to tailor their messages and offerings to each segment, resulting in more targeted and effective marketing campaigns.
4.9 out of 5
Language | : | English |
File size | : | 3286 KB |
Text-to-Speech | : | Enabled |
Screen Reader | : | Supported |
Enhanced typesetting | : | Enabled |
Print length | : | 256 pages |
Lending | : | Enabled |
Paperback | : | 56 pages |
Item Weight | : | 7 ounces |
Dimensions | : | 8.5 x 0.13 x 11 inches |
X-Ray for textbooks | : | Enabled |
Another area where machine learning excels is in predictive analytics. By analyzing historical data, machine learning algorithms can make accurate predictions about future customer behavior. For example, a retailer might use machine learning to predict which customers are most likely to make a purchase in the next month, allowing them to target their marketing efforts towards those individuals.
The Role of Deep Learning in Marketing
Deep learning is a subset of machine learning that focuses on developing neural networks with multiple layers. These networks are designed to mimic the human brain's structure and can learn complex patterns and relationships in the data. Deep learning algorithms are particularly effective in tasks such as image recognition, natural language processing, and sentiment analysis.
One of the key applications of deep learning in marketing is sentiment analysis. By analyzing customer reviews, social media posts, and other text data, deep learning algorithms can determine the sentiment associated with a product or brand. This allows marketers to gauge public opinion and make informed decisions about their marketing strategies.
Deep learning is also revolutionizing the field of image recognition in marketing. Brands can use deep learning algorithms to automatically identify and analyze visual content such as logos, products, and objects in images or videos. This opens up new opportunities for targeted advertising and personalized experiences.
The Benefits of Marketing Analysis and Prediction with Machine Learning and Deep Learning
The use of machine learning and deep learning in marketing analysis and prediction offers several benefits to businesses:
- Improved Efficiency: Machine learning and deep learning algorithms can process and analyze large volumes of data in a fraction of the time it would take a human analyst. This leads to more efficient decision-making processes and faster response times to market trends.
- Increased Accuracy: Machine learning and deep learning models can uncover subtle patterns and relationships in the data that human analysts may overlook. This results in more accurate predictions and better insights into consumer behavior.
- Better Personalization: By understanding customer preferences and behavior, marketers can personalize their offerings and messages, leading to higher customer satisfaction and loyalty.
- Cost Savings: By targeting marketing efforts towards the most promising customers, businesses can optimize their marketing budgets and reduce unnecessary expenses.
Marketing analysis and prediction using machine learning and deep learning have the potential to revolutionize the way businesses understand and engage with their customers. The ability to process and analyze large volumes of data, identify patterns, and make accurate predictions enables marketers to make informed decisions and stay ahead of the competition. As technology continues to advance, the role of machine learning and deep learning in marketing will only become more significant, leading to more personalized and effective marketing strategies.
4.9 out of 5
Language | : | English |
File size | : | 3286 KB |
Text-to-Speech | : | Enabled |
Screen Reader | : | Supported |
Enhanced typesetting | : | Enabled |
Print length | : | 256 pages |
Lending | : | Enabled |
Paperback | : | 56 pages |
Item Weight | : | 7 ounces |
Dimensions | : | 8.5 x 0.13 x 11 inches |
X-Ray for textbooks | : | Enabled |
This data set was provided to students for their final project in order to test their statistical analysis skills as part of a MSc. in Business Analytics. It can be utilized for EDA, Statistical Analysis, and Visualizations.
Following are the features in the dataset: ID = Customer's unique identifier; Year_Birth = Customer's birth year; Education = Customer's education level; Marital_Status = Customer's marital status; Income = Customer's yearly household income; Kidhome = Number of children in customer's household; Teenhome = Number of teenagers in customer's household; Dt_Customer = Date of customer's enrollment with the company; Recency = Number of days since customer's last purchase; MntWines = Amount spent on wine in the last 2 years; MntFruits = Amount spent on fruits in the last 2 years; MntMeatProducts = Amount spent on meat in the last 2 years; MntFishProducts = Amount spent on fish in the last 2 years; MntSweetProducts = Amount spent on sweets in the last 2 years; MntGoldProds = Amount spent on gold in the last 2 years; NumDealsPurchases = Number of purchases made with a discount; NumWebPurchases = Number of purchases made through the company's web site; NumCatalogPurchases = Number of purchases made using a catalogue; NumStorePurchases = Number of purchases made directly in stores; NumWebVisitsMonth = Number of visits to company's web site in the last month; AcceptedCmp3 = 1 if customer accepted the offer in the 3rd campaign, 0 otherwise; AcceptedCmp4 = 1 if customer accepted the offer in the 4th campaign, 0 otherwise; AcceptedCmp5 = 1 if customer accepted the offer in the 5th campaign, 0 otherwise; AcceptedCmp1 = 1 if customer accepted the offer in the 1st campaign, 0 otherwise; AcceptedCmp2 = 1 if customer accepted the offer in the 2nd campaign, 0 otherwise; Response = 1 if customer accepted the offer in the last campaign, 0 otherwise; Complain = 1 if customer complained in the last 2 years, 0 otherwise; and Country = Customer's location.
The machine and deep learning models used in this project are K-Nearest Neighbor, Random Forest, Naive Bayes, Logistic Regression, Decision Tree, Support Vector Machine, LGBM classifier, Gradient Boosting, XGB classifier, MLP classifier, and CNN 1D. Finally, you will plot boundary decision, ROC, distribution of features, feature importance, cross validation score, and predicted values versus true values, confusion matrix, learning curve, performance of the model, scalability of the model, training loss, and training accuracy.
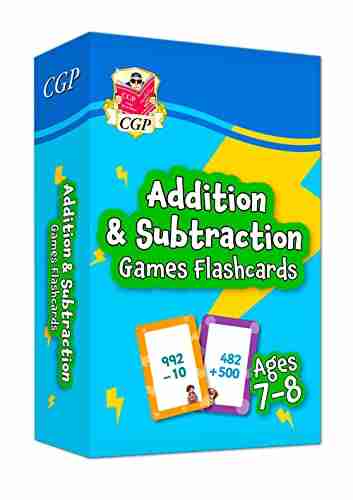

The Ultimate Guide to New Addition Subtraction Games...
In this day and age, countless parents are...
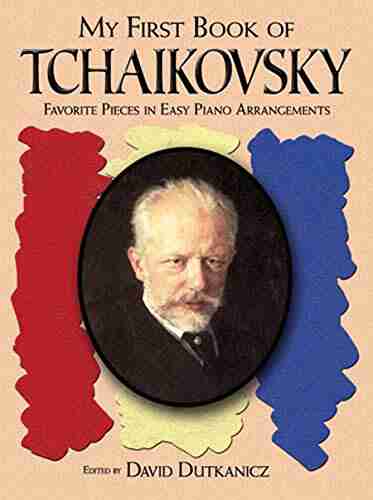

The Ultimate Guide for the Aspiring Pianist: Unleash Your...
Are you a beginner pianist feeling...
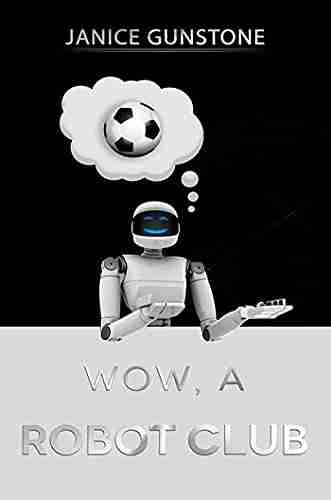

Wow Robot Club Janice Gunstone - The Mastermind Behind...
Robots have always fascinated...
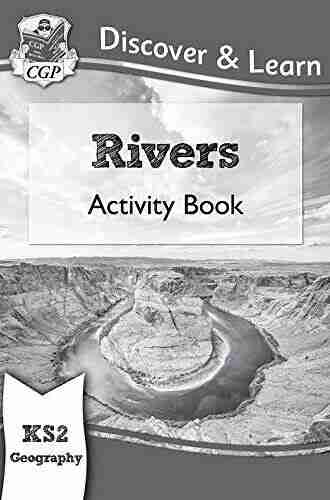

Ideal For Catching Up At Home: CGP KS2 Geography
Are you looking for the perfect resource to...
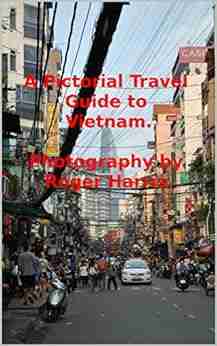

The Ultimate Pictorial Travel Guide To Vietnam: Explore...
Discover the rich...
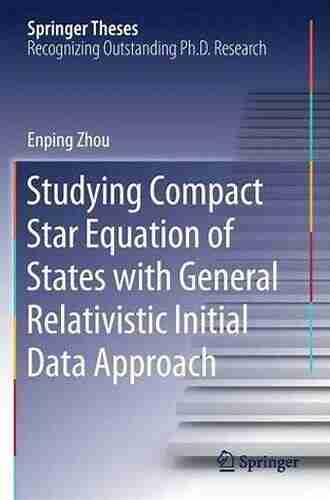

Unlocking the Secrets of Compact Stars: Exploring...
Compact stars have...
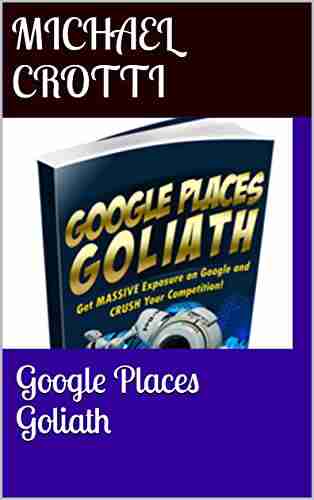

Unveiling the Hidden Gem: Google Places Goliath Valley...
Are you tired of visiting the same old...
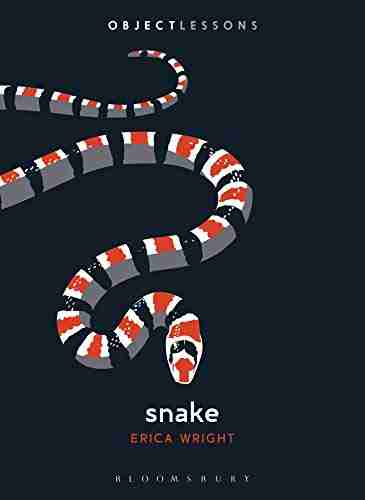

Essays Towards Theory Of Knowledge: Exploring the Depths...
Are you ready to delve into...
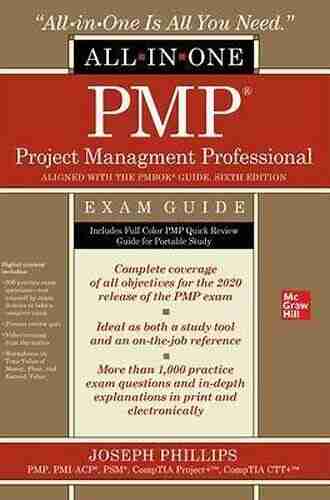

The Ultimate PMP Project Management Professional All In...
Are you ready to take your project...
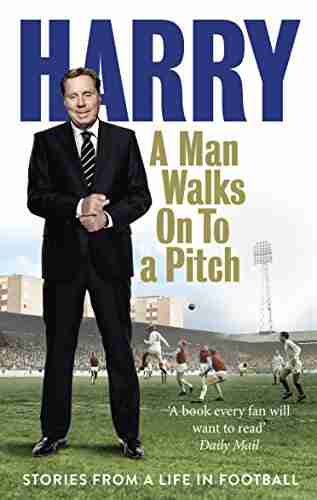

10 Incredible Stories From Life In Football That Will...
The Beautiful Game - Football...
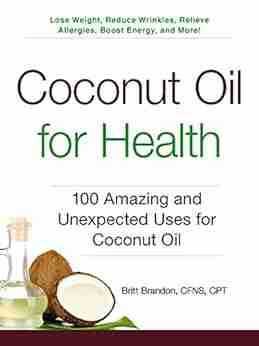

100 Amazing And Unexpected Uses For Coconut Oil
Coconut oil, a versatile and widely loved...
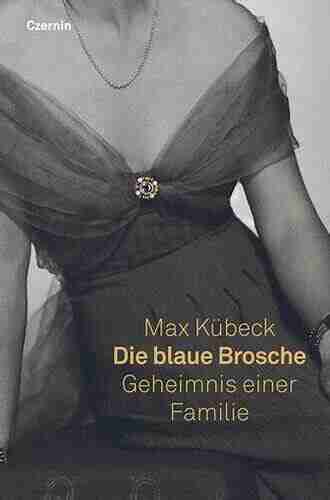

Unveiling the Enigma of Die Blaue Brosche: A Family’s...
Have you ever heard of Die Blaue Brosche...
Light bulbAdvertise smarter! Our strategic ad space ensures maximum exposure. Reserve your spot today!
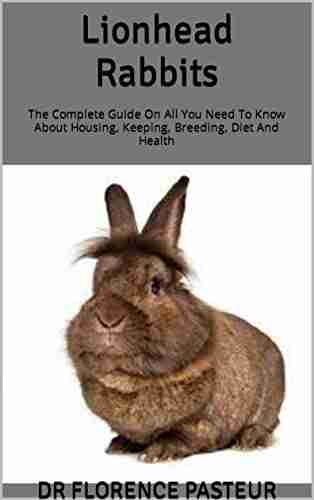

- Gil TurnerFollow ·12.1k
- Jack PowellFollow ·2.4k
- Stephen FosterFollow ·18.4k
- Sam CarterFollow ·14.8k
- Corbin PowellFollow ·2.5k
- Tyrone PowellFollow ·5.6k
- George MartinFollow ·12.3k
- Chance FosterFollow ·3.1k